 |
Brain Dynamics:
The Mathematics of the Spike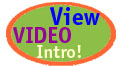
Every second, THIS
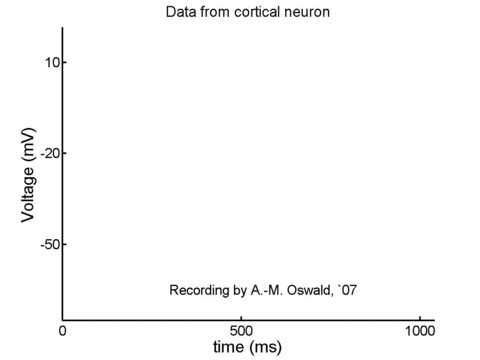
happens more than 100 billion times in your brain. You are seeing SPIKES, sudden electrical
impulses, shot through one brain cell on its way to the next. Spikes are the currency
of information in the brain and they drive everything we think and do. There are two basic
questions that brain science must answer: how are spikes formed and what do they mean?
Unraveling how neurons spike is a crown jewel of twentieth century neuroscience and mathematics
was central to this resolution. Resolving the second question, that is, understanding
the neural code, will be a central focus of twenty-first century neuroscience and mathematics
will surely contribute to this resolution as well.
A critical challenge that is not yet fully answered is to understand how spikes emerge from
tiny neurons, hundreds per pinhead. How is it that spikes are produced by a huge variety of
cells that look wildly different? How can different patterns of spikes be turned on and off in
a single cell in normal operation or through medicines? And since vastly different inputs
produce the same spike, just how does a neuron decide when to spike?
These questions have gripped the scientific community ever since spikes were
first seen more than 100 years ago. Hodgkin and Huxley, two physiologists,
showed how mathematics could solve all of them at once, laying the groundwork
for their 1963 Nobel Prize and for modern neuroscience. Today, mathematicians
are still building on Hodgkin and Huxley's theory of the spike to forge ahead
in brain science.
Follow the links to the right to see how nonlinear mathematics provides the
framework that unlocked the secret mechanics of the neuron. You’ll discover
how this mathematical framework is a nexus for modern neuroscience, and meet
Hodgkin and Huxley, the scientists who discovered it – and won a Nobel
Prize.
|
About the Authors
Eric Shea-Brown, Assistant Professor, Department of Applied Mathematics, University of Washington
Eric Shea-Brown is an assistant professor at the University of Washington in the Department of Applied Mathematics. His interests span a wide set of topics in mathematical neuroscience; current and recent projects focus on timing and decision making in idealized neural network models and on basic properties of correlations and reliability in spiking neural circuits. He and his colleagues are working to bridge the gap between these scales of modeling. Before UW, Shea-Brown was a postdoctoral fellow at NYU's Courant Institute and Center for Neural Science, and was mentored by John Rinzel. In 2004, he completed his PhD in Princeton's Program in Applied and Computational Mathematics where Phil Holmes and Jonathan Cohen advised him. They worked on stochastic neural network models, asking how they might be controlled to explain fascinating data from brain recordings and task performance.
WEB: http://www.math.nyu.edu/~ebrown/
CONTACT: ebrown@math.nyu.edu
Brent Doiron, Assistant Professor, Department of Mathematics, University of Pittsburgh
Brent Doiron is an assistant professor at the University of Pittsburgh in the Department of Mathematics. He earned his PhD in physics at the University of Ottawa where his interest in the intersection of mathematics and neuroscience began. He then spent three years as a postdoctoral fellow at New York University, living in Greenwich Village (meaning that a career in math can put people in some pretty cool places). Doiron now lives in Pittsburgh with his wife, who incidentally is also a neuroscientist, making it a family affair.
|
|
 |
|
 |